S2 #8 'How do we unravel the true climate-cost of AI and ChatGPT?', with Kasper Ludvigsen
Season 2 Episode 8
===
[00:00:00] James Gill: Hi there. Welcome to another episode of the EcoSend podcast. I'm your host James, and I'm thrilled to be giving you another show all about the climate and how that intersects with what we're doing in the digital and tech world. And this week we are talking about AI. And if you've been at all close to the internet as most of us are, you'll probably struggle to go even an hour without hearing something about AI, machine learning ,ChatGPT these days.
[00:01:23] AI and all of the surrounding technologies there supposedly have the power to change our lives enormously, or end all civilization. And so we'll have to find out how that plays out. But it's a topic we care about very deeply here at EcoSend because we are all about trying to build the best email marketing software on the planet.
[00:01:45] And we also care deeply about the climate and sustainability. And AI plays a huge part in both of those sides for positives and negatives. And so there's a lot of questions and perhaps not enough conversation going on right now about the climate cost of AI and these and these systems. So I am very excited to be speaking with someone who knows a bit about this subject.
[00:02:12] And that is Kasper who joins me today. Kasper is a data scientist at the Danish National Police with several years of experience in consulting in the financial sector. Kasper is also co-founder and board member at the Danish Data Science Community. The D D S C Kasper cares about reducing the environmental impact of software in general and data science in particular.
[00:02:36] So makes a great candidate to talk to about this and especially things like reducing the carbon footprint of training and serving machine learning models. So I think you could be the most perfect person to be speaking to about this topic. Kasper, welcome to the show. How are you doing today?
[00:02:51] Kasper Ludvigsen: Thank you very much, James. I'm well and I'm very happy to, to be on the show because this is a topic that that is very dear to me.
[00:03:00] James Gill: Absolutely. I, I am very keen to unpack a lot of things here. I think many of us are struggling to even keep up with whatever today's News is, or even last week's News is about AI and whatever new thing has come out. And by the time this show airs, which usually is only a few days to a week after we record it, there'll probably be something new come along.
[00:03:23] But I am very keen to talk about about yourself first and how you got into the world of both AI machine learning, probably the hottest thing going right now, but also why you care about sustainability and the climate too. I'm keen to hear everything
[00:03:41] Kasper Ludvigsen: Yeah. Thanks. Yeah, so how I got into AI all started, I think it was back in 2015 when I read a blog post from a blog called, 'waitbutwhy'', which detailed how AI would revolutionize basically everything; all aspects of society. And it also referenced how some scientists speculated that in the relatively near future we would have AI that would be as intelligent as humans.
[00:04:19] And I thought that is very exciting and it sounds like something that I want to be a part of. And so I, that's how I began my, my journey into, into machine learning. Because that's what I think of when I think of AI, and at some point my interest in machine learning or AI, let's call it AI for now, because that's that's a term that has a bit of a broader appeal.
[00:04:46] So my interest in AI intersected with my interest in sustainability when I realized at some point that it, like making or developing AI models requires a lot of energy. And given that not all our energy is from renewable sources; training these models or developing these AI models has a carbon footprint that is sometimes noticeable if I were to use a rather neutral term.
[00:05:21] James Gill: Yes.
[00:05:22] Kasper Ludvigsen: So yeah, I'll attempt to, or try to not be not to offer too many value judgments when I speak about this topic because I'd rather focus on the numbers and maybe let other people make, make their own decisions about what they believe is right or wrong in, in this debate. But, yeah.
[00:05:45] So my realization of the, of the unforeseen environmental impacts of AI started to... you know, this realization came to be when I read a few research papers. One of them was by Emma Strubell. She's an American researcher. She wrote a paper that's titled something along the lines of ' Policy considerations for large scale natural language processing' or, or something along those lines.
[00:06:17] And another paper simply called 'Green AI' which were at least to my knowledge, some of the first academic papers that addressed the, the environmental impacts of, of AI specifically the carbon footprint of large language models.
[00:06:36] James Gill: When were those, when were those written, out of interest?
[00:06:41] Kasper Ludvigsen: I think the, the Emma Strubell one was in 2019, but I didn't come across it. I think I came across it in 2020. Yeah. And then I, I also simultaneously, Like, as I started to think about these issues, I also, it also became apparent that not a lot of people talked about it, at least not, you know, just a few years ago, not very many people talked about these issues.
[00:07:16] And I thought it would be meaningful for me to partake in this journey towards broader awareness of the environmental impacts of AI. And that's that's also when I started to write about these things and, and talk to people like you and give presentations and these types of activities.
[00:07:41] Yeah and generally, I think that climate change is is one of the most meaningful problems to to, to address for me personally and given that I am, that I don't address this problem directly in my day-to-day work I found a very meaningful spare time activity to talk about these things.
[00:08:09] James Gill: By by day, thinking about machine learning by night, saving the world. Yeah.
[00:08:13] Kasper Ludvigsen: Catching crooks with code, as I sometimes say at day. And then writing blog posts at night.
[00:08:21] James Gill: Yeah. Now I know you have written extensively on the topic Kasper. I'm sure we'll link in the show notes of the episode to some of your, your articles on the topic of ChatGPT of AI and the carbon footprint there. Maybe listeners who are following along and myself included here, sometimes there is a lot to understand with, with what we even talked about when we talk about AI and and, and especially with ChatGPT talked about large language models there. Is there a, like a real beginner's intro to what we're actually talking about here and, and why this uses energy more so than say a standard webpage loading on the internet? I, it would be really interesting to hear your take. And I, I would also, I know we have we try to keep these shows to half an hour. I'm sure you could talk all day about this Kasper. But if you do the magic and condense it down into a few minutes, that would be amazing.
[00:09:29] Kasper Ludvigsen: Yeah. Sure, sure.
[00:09:30] James Gill: That's a tough ask I know!
[00:09:31] Kasper Ludvigsen: Yeah. So it's, it's my, my impression that there's no universal definition of what AI is as opposed to machine learning and deep learning and large language models and so on. But the way I like to think about it is that AI is a sort of umbrella term. So that's AI is more or less making computers behave intelligently.
[00:09:51] And for now, let's just ignore what it means to behave intelligently. But then you have different methods for achieving intelligent behavior by computers. And one of these in my opinion, one of these again, umbrella terms that's below AI, that's machine learning. And then you have different types of machine learning one of them being deep learning.
[00:10:18] And then the way you do deep learning is you build a very deep narrow network architectures. And deep in this context means that these neural network architectures have several layers where each layer performs some sort of computation. And then once you finish training; once you finish a training procedure you end up with a deep learning model that could be a large language model and large language models is basically a type of deep learning or machine learning model.
[00:10:55] That to put it very simply can do things with text data.
[00:11:00] James Gill: Right.
[00:11:01] Kasper Ludvigsen: yeah. Yeah, some say that ChatGPT is basically just predicting the most likely next word.
[00:11:09] James Gill: Sure. Like predictive text on your phone. Yeah,
[00:11:12] Kasper Ludvigsen: yeah. So when , when you ask the question to put very simply again, what it does is it generates a word and then it predicts the most likely next word in its response and so on.
[00:11:23] And that's probably too simplistic, but that's how some people conceptualize what it is that ChatGPT is doing. And now to the question, why do these things have a carbon footprint? Or why do these things have an environmental impact? That is because they are a type of digital service and all digital services, they need to run on some computer hardware.
[00:11:51] And all computer hardware requires electricity to run and all electricity or, well, yeah. This is something that is maybe out of scope for this episode, but some people would probably say that all electricity has a carbon footprint, even the electricity from renewable sources because you could in theory divide or like distribute the initial carbon footprint of a solar panel park.
[00:12:23] You could distribute those environmental costs across all the electricity units generated by that solar panel park. So that's why some people say that even renewable energy has a carbon footprint. But anyway, now I'm probably going into too much detail
[00:12:39] James Gill: No, that is, no, no, that is very, very interesting. No, no.
[00:12:42] Kasper Ludvigsen: So that is why these models can have a carbon footprint to begin with.
[00:12:47] And the reason that the carbon footprint is sometimes noticeable is that especially large language models like the ones that power services like they require huge amounts of data to achieve their abilities. And every time they look at a piece of data during their training process or during the inference process, that data has to pass through some very com complex computations, or at least some very energy intensive computations. So these large models, they're just much more energy intensive than, you know, the very simple models that you may use to predict the price of a home or some other, like relatively simple machine learning use case.
[00:13:41] Yeah. And so when you, when you think about the environmental impact of these models it makes sense sometimes to think about different stages of an AI system's lifecycle. And one way to conceptualize the stages of an AI systems lifecycle is to say that first you have some materials extraction, and then you have some materials manufacturing, and then you have hardware manufacturing.
[00:14:10] And then you have model training, which is where you train the model to learn to do a specific task. And this process, if you were to make an analogy to web development, this process would be similar to the process you go through to code the website. Then the next stage in the lifecycle would be the so-called deployment stage, which is where the trained AI model is made available for its users.
[00:14:39] And this is the stage that ChatGPT is currently in. And, and again, going back to the website analogy, that would be when you are done coding your website and now it's live for people to see.
[00:14:52] Yeah. And then the final stages, then the end of life where you dispose of, for instance, the hardware on which your AI system has been running.
[00:15:01] And yeah, and, and then obviously the carbon footprint or the environmental impact differs between these different stages.
[00:15:10] James Gill: Sure. That's actually fascinating because I guess we talk a lot about the energy usage of, say the servers running. Like you imagine that server, that computer in a dark data center somewhere with a fan spinning away. But you're considering also all of the supply chain, I guess, of putting that server in that data center.
[00:15:33] And then what happens with that after it's done, after a grueling life processing all these calculations.
[00:15:40] Kasper Ludvigsen: Exactly. And so when you, when you use ChatGPT, obviously, the device that you are using to access ChatGPT, that device also has a sort of a carbon footprint or an environmental impact. There's some electricity consumption associated with powering your computer. But that's completely different from what I'm normally talking about when I talk about the environmental impact of an AI system.
[00:16:07] When I talk about that, I'm, I'm only referring to the the actual environmental costs associated with developing and making this type of service available.
[00:16:18] James Gill: Sure, sure. I guess the computer I'm using to access that ChatGPT has like a whole different set of conditions and I might be using that for all sorts of different things, so, yeah.
[00:16:32] Kasper Ludvigsen: Yeah, exactly. And if you're a company and you want to, you know compute your total carbon footprint. Those two are two very different things.
[00:16:44] James Gill: Hmm. Absolutely. Okay, so, so this, this is making a lot of, I think it's making a lot of sense. Kasper, you're doing a very good job of explaining an incredibly complex topic So, ChatGPT is an example of one of these large language models and it's clearly exploded in popularity since its release to the, into the wild, like almost like the dinosaurs at Jurassic Park running, running wild.
[00:17:14] What has that meant for sort of the energy consumption? Does that mean there's a lot more servers that have been needed and a lot more energy that's been needed to power those servers? And how does one even go about establishing how much energy that might be, let alone the carbon footprint of that?
[00:17:33] Kasper Ludvigsen: Yeah, well, that's not at all an easy task. And the main reason that it's a very difficult undertaking is that OpenAI and other providers of, of these types of services for that matter. They don't publish the data that we need to make these sort of assessments in you know, in the ideal world, OpenAI, they would themselves publish, for instance the energy consumption associated with asking ChatGPT one question.
[00:18:09] But, but even if they did, that number would depend on some assumptions such as, for instance, the average length of the question that you ask and the average length of the response that you get. So, so even if OpenAI gave us these numbers they would have to rely on some assumptions.
[00:18:34] Obviously they could perhaps just measure the total energy consumption from running the service that host ChatGPT and then divide that total energy consumption by the total number of queries.
[00:18:51] That would give you an, an indication of the average energy consumption per query. But if you are let's say you're a business and you want to know how many or how, how big a carbon footprint does our use of ChatGPT have, then you would be relying on an average provided by OpenAI and, and not the actual energy consumption associated with the questions that you ask.
[00:19:16] So it's a very complex topic, but I've written a few blog posts where I provide some estimates. That's, that's how I like to refer to them.
[00:19:26] I try to, I try to arrive at an estimate of what could the carbon footprint and electricity consumption of ChatGPT be.
[00:19:34] And one way that I initially went about it was I looked into the research literature and I found a paper by a researcher from 'Hugging Face' called Sasha Luccioni. And she has some co-authors of this paper where they they estimate the lifecycle carbon footprint of the language model that is called 'Bloom'.
[00:19:57] And this language model is interested in estimating the carbon footprint of ChatGPT. Because Bloom and ChatGPT's underlying models are very similar in their size. And so this paper by Luccioni and others they found that the average energy consumption or electricity consumption per query to Bloom was 0.0039 kilowatt hours.
[00:20:30] And so I used that as a starting point, and then I made some estimates, or, you know, I made some assumption about how many users do we think that ChatGPT may have, and you know, then simply by multiplying the energy consumption per query by the total number of queries that we think ChatGPT receives allows us to at a guesstimate.
[00:20:55] But the issue with this approach is that even though Bloom and ChatGPT;s underlying model are similar in size it's fair to assume that OpenAI has probably put an effort into optimizing ChatGPT's underlying model. Because the more efficient it is at processing your questions the better the user experience because the less you have to wait for a response, but also the fewer costs they accrue from hosting this service.
[00:21:29] And then also coincidentally, maybe I should give them the benefit of doubt, but I don't think that their motivation for this is to reduce their energy consumption, but coincidentally, this would probably also reduce their energy consumption.
[00:21:43] James Gill: Right. Yeah. So the aligned interests there of reduced costs.
[00:21:49] Kasper Ludvigsen: Yeah. yeah. So that's one positive aspect of this that usually everything else held equal, if your model is more efficient like if it can process requests quicker, it also uses less energy.
[00:22:03] Well simply put, I'm sure there can be cases where this is not true, so then to use a different method for estimating the carbon footprint and electricity consumption of ChatGPT, I used some other people's estimate of how many GPUs are needed to run the ChatGPT service. So this is an article by some guys whose last names are Patel and Ahmed, if I remember correctly. But they, they wrote a really nice article that their purpose was not to estimate the Environmental costs, but the monetary costs of running the ChatGPT service. So they estimated many GPU's you need to serve ChatGPT to the number of users that we think ChatGPT has. And they, they estimated that you need roughly 29,000 GPUs.
[00:23:01] And for those who are not familiar with the hardware component GPU, it's basically data centers use it to run AI models, it's a piece of hardware that's optimized for running AI models. And it's more efficient usually and quicker than other types of computer processes. But, but yes, it's 29,000 of these. That's a huge amount,
[00:23:26] James Gill: That is a, a lot of, a lot of GPUs.
[00:23:30] Kasper Ludvigsen: When you buy a consumer laptop that you can use for gaming it it has one GPU, and that GPU is very, very weak compared to the data center GPUs. So imagine that you need 29,000 laptop GPUs, but you need them to be like 10 times or like thousands of times more powerful than your laptop GPU.
[00:23:52] Anyway, so that's, it's just a huge amount of GPU. But yeah, so I, I said, okay, these guys say they seem quite knowledgeable about hardware. I think their estimate is is about right. So if we think ChatGPT requires 29,000 GPUs, we can actually compute the total energy consumption from these GPUs.
[00:24:15] If we make an assumption about how much electricity these GPUs use as a ratio of their maximum electricity usage because you can actually on the internet Nvidia, the manufacturer of these GPUs, they actually do provide information about what's the maximum power draw of these GPUs. So if I remember correctly, the maximum power draw of one GPU is 0.4 kilowatts.
[00:24:43] That means if, if this GPU runs for one hour, it will have consumed 0.4 kilowatt hours of electricity.
[00:24:53] Then you know, you you need to make an assumption of is it running at full power all the time or is it running at maybe 75% or 50%? So let's say it's running at 50% of maximum power draw.
[00:25:06] Then you have in one hour, this one GPU will have consumed 0.2 kilowatt hours, which means that you have to multiply 0.2 kilowatt hours by 29,000. Then you arrive at the hourly energy consumption from all these GPUs which you can then multiply by 365 to arrive at the annual electricity consumed from these GPUs.
[00:25:30] So, but yeah.
[00:25:32] James Gill: It's fascinating hearing the I, I am being crude by saying back of envelope maths, but you know...
[00:25:40] Kasper Ludvigsen: it is!
[00:25:41] James Gill: It, it's amazing what you can find from well, especially from an organization called OpenAI. They're not being exactly very open about some key, key info here.
[00:25:51] Kasper Ludvigsen: Okay. Okay. If I can just make one point. So everything I just said assumes that ChatGPT is running on the language model called GPT 3.5 because I did these estimates a few months back. But now OpenAI has released an even bigger or reportedly an even bigger model GPT four.
[00:26:15] And it's rumored to have 1 trillion parameters. The number of parameters is just a way to, to talk about the size of the model. And GPT3 had 175 billion. So GPT4 is a bit more than five times larger than its predecessor. So we, I think it's safe to assume that ChatGPT now has a bigger energy consumption per query than it used to have if we think that ChatGPT is now running on GPT4. So that was the electricity consumption. If, if I can just briefly touch upon the carbon footprint; that's a whole other ballgame that's really, really difficult because now ChatGPT is so widely used that it's very safe to say that it's running in multiple centres across the world.
[00:27:16] And each geographical region around the world has their own carbon footprint per electricity unit that you consume. And this is because some countries they depend a lot on coal and some depend, or some have a lot of renewable energy, so, as everyone is probably aware some countries have rather clean energy and some have energy that's less clean.
[00:27:41] And so if you wanted to compute the total carbon footprint of ChatGPT, you would need to know specifically what data centres the model is running in and how many queries each data centre handles. And then you could probably find data about the average carbon intensity of electricity of that specific region.
[00:28:07] But this is something like those two first things I mentioned, there's no way we can know this, so it's not feasible really to make any I don't know, guess about the carbon footprint. But what you can do is if you're a, if you're a business and you would like to know your own carbon footprint from using ChatGPT, for instance, as part of your email marketing automation efforts, then you could use any of my estimates of the energy consumption per query.
[00:28:44] And then you have to track how many queries do you send to ChatGPT, and then you would have to make an assumption about what data centre you think enters your requests. So you guys, you're you're based in the UK so that's probably a data center in Ireland. And so you could use the average carbon intensity of electricity produced in Ireland to arrive at your total carbon footprint from using ChatGPT.
[00:29:19] Yeah. So it is possible, you know, at the granular level to arrive at estimates of the carbon footprint that are reasonable or sensible, but on the larger scale yeah, you would have to probably just use the global carbon intensity of energy. If you wanted to, you know, try and compute the total carbon footprint of chat.
[00:29:42] Because there's, there's no way you can know all the details you need to know in order to make a granular estimate.
[00:29:48] James Gill: Absolutely. I guess for, for many people, especially in a software background, it's one of those things that everyone's so used to having very precise calculations and measurement for things. And then when it comes to this, you're dealing with so many variables and it's impossible to get to the absolute perfectly measured result, isn't it?
[00:30:09] Kasper Ludvigsen: Yeah, that's, that's so true.
[00:30:12] James Gill: Yeah. I know. I know we're almost at time, but I know that one of the things you did also mention was we talk a lot on this subject of measurement. We talk a lot about carbon footprint, but I know you are also saying that there's other aspects around water usage and air pollution and, and even soil pollution as well...
[00:30:32] Kasper Ludvigsen: Yeah. That's soil contamination. Yeah.
[00:30:34] James Gill: Yeah. Briefly, if you can, Kasper, what's the impact of those, because those are, you know, beyond any of the stuff we've even just talked about, right?
[00:30:44] Kasper Ludvigsen: Yeah. I think I'm not the only one who failed to give attention to these other environmental impacts. Because I have, I've struggled to find you know, data or information that I can work with to estimate those other impacts except for the water consumption or the water footprint.
[00:31:08] Because recently there was a scientific paper that even made it to mainstream media which estimated the water footprint of some AI or one AI model. I can't remember which one. But on a side note, they said that ChatGPT probably consumes a half a litre of water per 20 to 50 queries. So let's say, you know, let's say that it's 50 queries takes half a litre, then it's 10 milliliters per query. That doesn't sound like a lot, but if you have billions of queries even each day then the water consumption will become noticeable.
[00:31:54] I recently did, did again some what did you call it? Back of the envelope maths. I like, I like that expression. It was just a brief LinkedIn post. Because I, I felt this was something that that required more attention. So I wrote a brief LinkedIn post about this topic and, through my back of the envelope maths, I estimated that ChatGPT may have consumed 250 million litres of water in April 2023. And then some people say, you mean reused? Because they assume that ChatGPT is being cooled down by, I don't know, wastewater or something. But no, that's not the case.
[00:32:42] It's actually water, clean water that could have been drunk, that is being used for cooling. The data centers that host ChatGPT. And then some, some people say, okay, well this water, it evaporates. So it's not really a problem because, you know, it just stays within, you know, earth's atmosphere. And I guess that's true.
[00:33:05] I'm not a physicist but let's just say that's true, but that's not the problem. The problem is that you're actually using water in regions that can be affected by drought. So in this research paper, the authors showed a map of the US which highlighted areas that are affected by drought at some interval.
[00:33:28] And they said that in these areas that are affected by drought you have thousands of data centers.
[00:33:36] So you know, in, in regions that, that don't have enough water. You do have data centers that consume potentially millions of liters of water. And I think that's that's another very important environmental impact of, of AI and of our digital world in general.
[00:33:58] That we, that we need to give more attention to.
[00:34:02] James Gill: That is absolutely fascinating because comparatively to people talking about carbon footprint and carbon offsetting like, the water side and how that relates to digital services is barely being spoken about. And, and I think there's something so tangible about saying this stuff that I'm drinking all day is being used up by the same things I'm writing on my computer.
[00:34:25] That is incredibly eye-opening. Kasper, I would love to talk to you for another... I would love to talk to you for the rest of the day! But I am afraid we must wrap out an absolutely fascinating treasure trove of an episode. So I want to make sure anyone listening can hear more, get in touch learn more from your words of wisdom and hear more of your back of envelope calculations, which I am certainly going to be looking for.
[00:34:55] So I believe people can find you on LinkedIn and on on Medium where you've been writing some phenomenal work. So we'll link both of those in the show notes.
[00:35:05] Kasper Ludvigsen: Yeah. Sounds good. And just wanted to let people know that you can feel free to connect even if LinkedIn says I prefer to be followed. And I have a few new blog posts in the pipeline on, on this topic. So stay tuned.
[00:35:19] James Gill: Amazing Kasper. Thank you. We'll definitely be looking out for those and yeah, we'll do our best to share those with more of the EcoSend audience because I'm sure people listening will be very keen to see those. So, Kasper it's been an absolute pleasure. Thank you for enlightening myself at the very least on, on a whole range of topics here.
[00:35:40] Kasper Ludvigsen: Thanks for inviting me. It was a pleasure.
[00:35:42] James Gill: Yes, thank you. No, it's been, been an absolute pleasure. And thank you to those of you who are listening and watching. We look forward to bringing you more shows in the future. If you've enjoyed Kasper's episode today, please do let us know via whatever podcast player you're using.
[00:36:00] And it always helps spread the word about about all of the wonderful guests that we have on this show. So thank you and we'll see you next time.
[00:36:08]
Creators and Guests
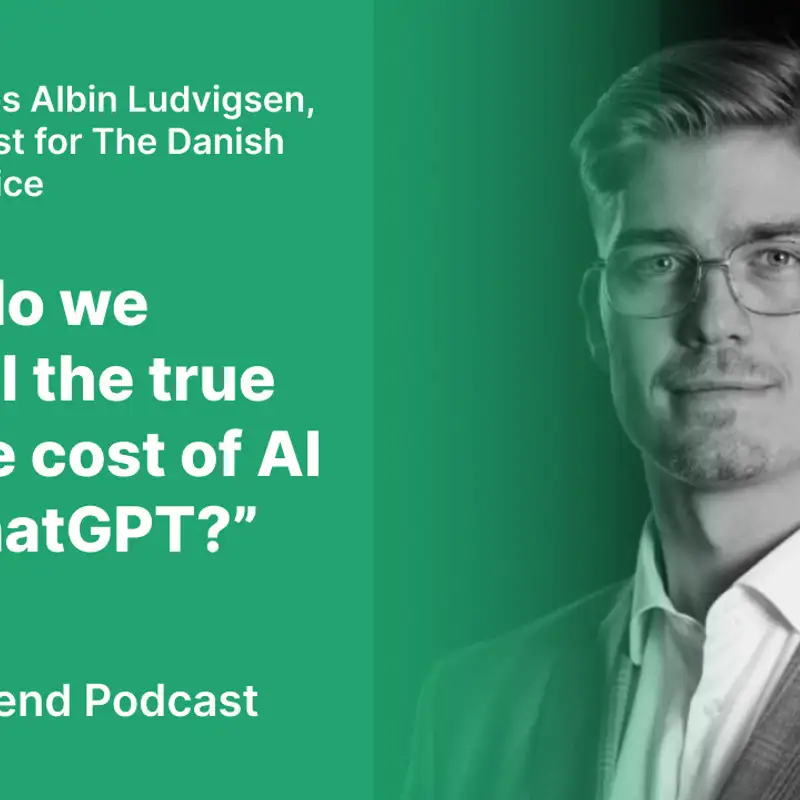